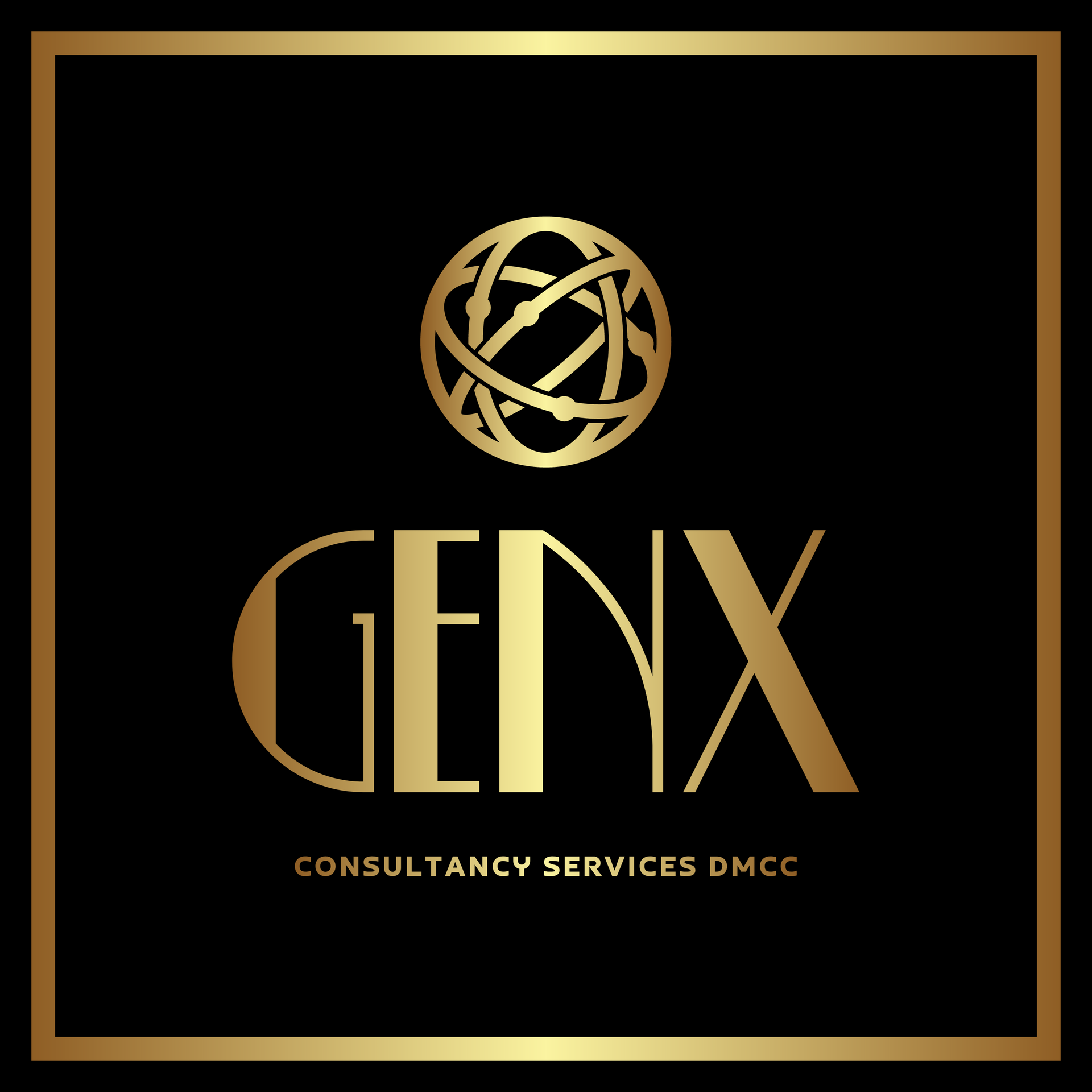
Streamlining data integration with Fivetran
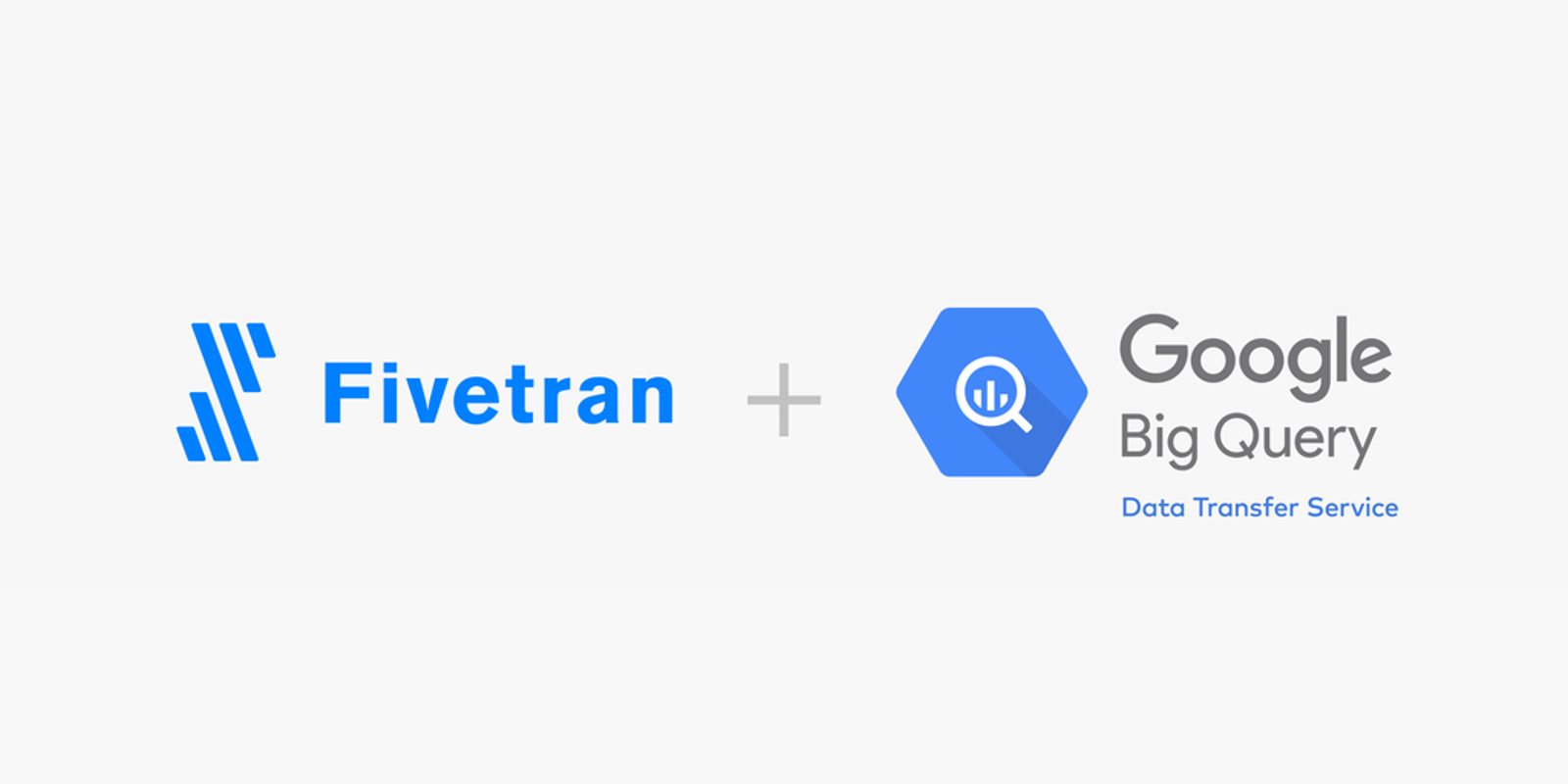
In today’s dynamic landscape, businesses need faster data analysis and predictive insights to identify and address fraudulent transactions. Typically, tackling fraud through the lens of data engineering and machine learning boils down to these key steps:
- Data acquisition and ingestion: Establishing pipelines across various disparate sources (file systems, databases, third-party APIs) to ingest and store the training data. This data is rich with meaningful information, fueling the development of fraud-prediction machine learning algorithms.
- Data storage and analysis: Utilizing a scalable, reliable and high-performance enterprise cloud data platform to store and analyze the ingested data.
- Machine-learning model development: Building training sets out of and running machine learning models on the stored data to build predictive models capable of differentiating fraudulent transactions from legitimate ones.
Common challenges in building data engineering pipelines for fraud detection include:
- Scale and complexity: Data ingestion can be a complex endeavor, especially when organizations utilize data from diverse sources. Developing in-house ingestion pipelines can consume substantial data engineering resources (weeks or months), diverting valuable time from core data analysis activities.
- Administrative effort and maintenance: Manual data storage and administration, including backup and disaster recovery, data governance and cluster sizing, can significantly impede business agility and delay the generation of valuable data insights.
- Steep learning curve/skill requirements: Building a data science team to both create data pipelines and machine learning models can significantly extend the time required to implement and leverage fraud detection solutions.
Streamlining data integration with Fivetran
In order to build effective fraud detection models, they needed all of their data to be:
- Curated, contextual: The data should be personalized and specific to their use case, while being high quality, believable, transparent, and trustworthy.
- Accessible and timely: Data needs to always be available, high performance, and offering frictionless access with familiar downstream data consumption tools.
The firm chose Fivetran notably for its automatic and reliable handling of schema evolution and schema drift from multiple sources to their new cloud data platform. With over 450 source connectors, Fivetran allows the creation of datasets from various sources, including databases, applications, files and events.
BigQuery’s scalable and efficient data warehouse for fraud detection
BigQuery is a serverless and cost-effective data warehouse designed for scalability and efficiency, making it good fit for enterprise fraud detection. Its serverless architecture minimizes the need for infrastructure setup and ongoing maintenance, allowing data teams to focus on data analysis and fraud mitigation strategies.
- Faster insights generation: BigQuery's ability to run ad-hoc queries and experiments without capacity constraints allows for rapid data exploration and quicker identification of fraudulent patterns.
- Scalability on demand: BigQuery’s serverless architecture automatically scales up or down based on demand, ensuring that resources are available when needed and avoiding over-provisioning. This removes the need for data teams to manually scale their infrastructure, which can be time-consuming and error-prone. A key part here to understand is that BigQuery can scale while the queries are running/in-flight — a clear differentiator with other modern cloud data warehouses.
- Data analysis: BigQuery datasets can scale to petabytes, helping to store and analyze financial transactions data at near-limitless scale. This empowers you to uncover hidden patterns and trends within your data, for effective fraud detection.
- Machine learning: BigQuery ML offers a range of off-the-shelf fraud detection models, from anomaly detection to classification, all implemented through simple SQL queries. This democratizes machine learning and enables rapid model development for your specific needs. Different types of models that BigQuery ML supports are listed here.
- Model deployment for inference at scale: While BigQuery supports batch inference, Google Cloud’s Vertex AI can be leveraged for real-time predictions on streaming financial data. Deploy your BigQuery ML models on Vertex AI to gain immediate insights and actionable alerts, safeguarding your business in real-time.
The combination of Fivetran and BigQuery provides a simple design to a complex problem — an effective fraud detection solution capable of real-time, actionable alerts. In the next series of this blog, we’ll focus on the hands-on implementation of the Fivetran-BigQuery integration using an actual dataset and create ML models in BigQuery that can accurately predict fraudulent transactions.a