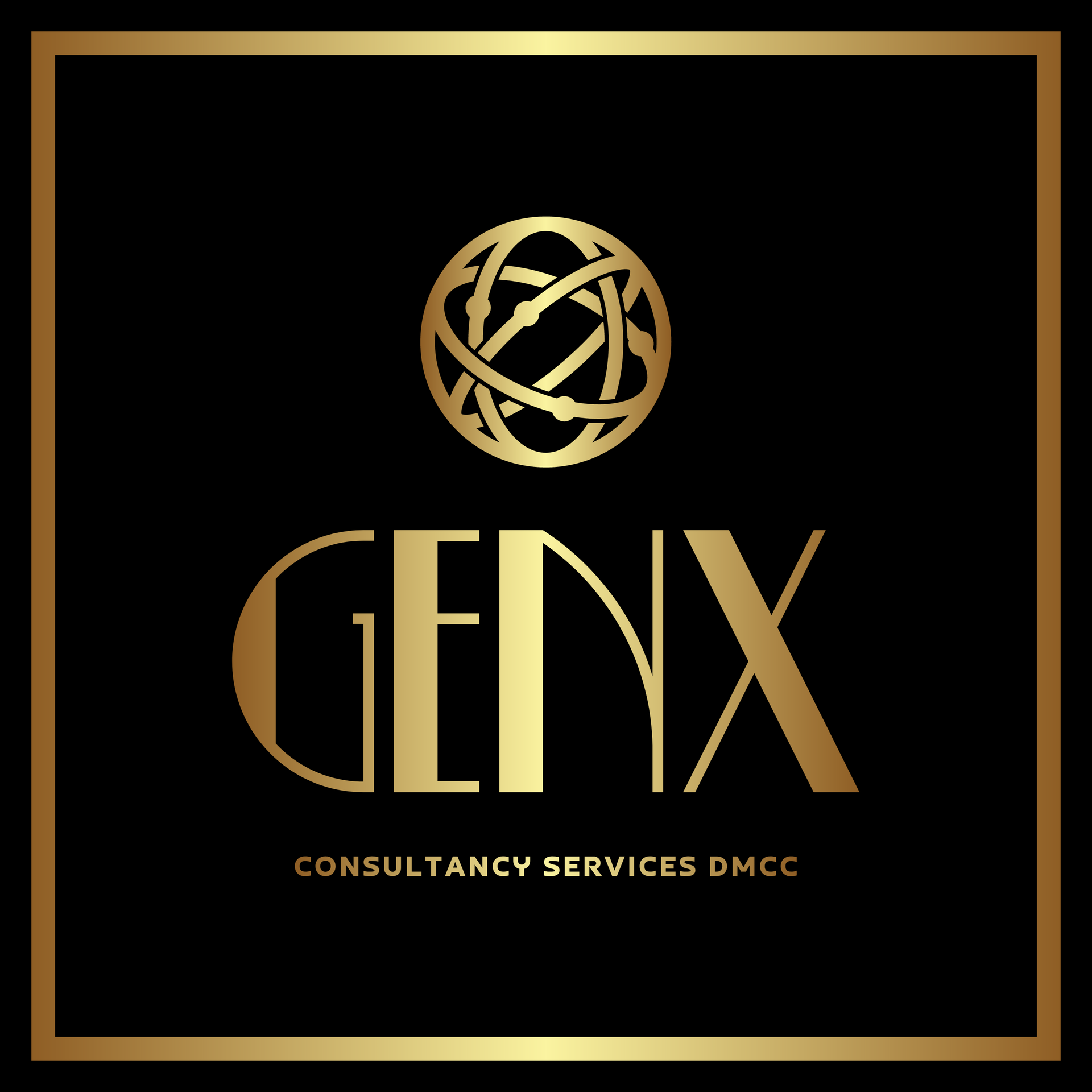
Role of Data Warehouse Automation in the Lifecycle
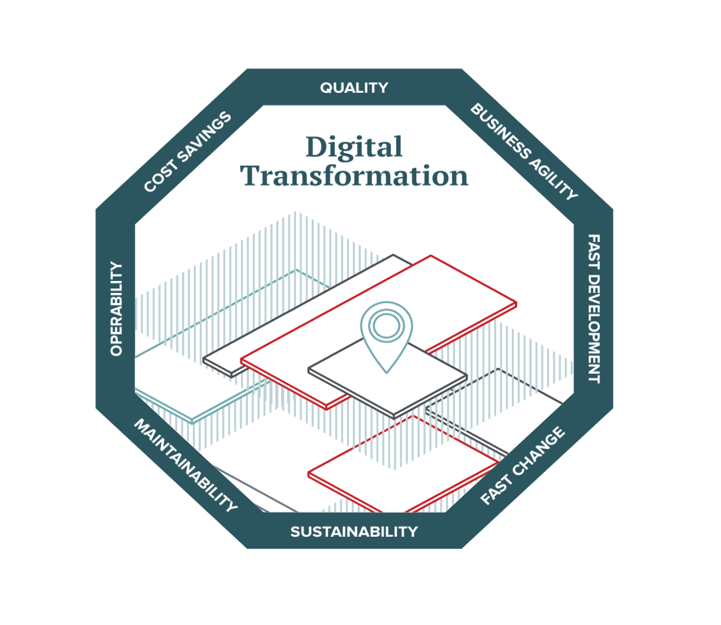
Data Warehouse Automation (DWA) refers to the process of automating various aspects of data warehouse development, deployment, and maintenance. It aims to streamline and accelerate the traditionally time-consuming and labor-intensive tasks involved in building and managing data warehouses.
A data warehouse is a way to provide business analysts and other users with a centralized repository of enterprise data from which to glean insights that guide business decisions.
What is Data Warehouse Automation?
Data warehouse automation (DWA) helps IT teams deliver and manage much more than before, much faster, with less project risk and at a lower cost by eliminating repetitive design, development, deployment and operational tasks within the data warehouse lifecycle.
The Data Warehouse Institute (TDWI) defines data warehouse automation as:
“…using technology to gain efficiencies and improve effectiveness in data warehousing processes.
Data warehouse automation
is much more than simply automating the development process. It encompasses all of the core processes of data warehousing including design,
development, testing, deployment, operations, impact analysis, and change management.”
With automated data warehousing, IT teams can fast-track new data integration, more effectively work with big data, and devote greater time to the business intelligence initiatives that will yield the greatest impact for their organizations.
Different Stages of the Lifecycle
The linear stages of the data warehouse lifecycle are listed below.
- Identify requirements
- Data modeling
- ETL/ELT development
- Set up OLAP
- UI development
- Maintenance
- Test & QA
- Deploy to Production
Role of Data Warehouse Automation in the Lifecycle?
Data warehouse automation can speed up and condense each lifecycle stage from weeks to minutes. With DWA, data processing undergoes ETL optimization methods to increase reliability.
Automation can streamline the entire data warehouse lifecycle. It is instrumental in the ETL stage, where huge amounts of data from multiple sources must be consolidated. Setting up ETL automation can significantly reduce the developmental complexities within a data warehouse development project.
Data Warehouse Automation Benefits
Data warehouse automation provides data warehousing teams with a wide range of benefits commonly associated with digital transformation as seen in the diagram above.
- Streamline Data Integration ETL Tools
- Data warehouse automation software facilitates the creation of reusable bidirectional connectors with data sources.
- With the ability to import data from different sources, be it files, databases, cloud apps, or any other data source, automation can help you take full advantage of no-code data mappings and ETL tools.
2.Build Machine Learning Models
- Data warehouse automation tools allow for the creation of dimensional models with ease, increasing the agility and efficiency of your data warehouse operations.
- Using machine learning algorithms can help identify and build these data models with minimal effort and time.
3.Increased Data Team's Productivity
- Engineers and analysts can focus on higher-priority requests by automating routine data management tasks.
- With DWA software, there's no need to search StackOverflow frantically for ETL scripts and manual data warehouse workflows.
4. Replicate Proven, Secure Data Marts
- Automation enables the designing of high-quality data model templates, reducing time and room for error.
- Audit real-time data to verify consistent data quality before connecting it to business intelligence visualization tools.
- 5.Improved Reporting and Analytics
Data warehouse automation software can increase the reliability of your data warehouse solution. - With up-to-date data, downstream reports and analysis will offer more actionable than disparate data providers.
6. Increase Regulatory Compliance
- Data pipelines can enforce proper data quality standards to meet privacy and security regulations in advance rather than as an afterthought.
- DWA providers such as WhereScape, Azure, and Amazon can help maintain compliance and achieve warehousing goals.
7. Integrate Data With Drag-and-Drop Workflows
- With drag-and-drop tools, creating a workflow for data lake processing, big data analysis, and other tasks can be more manageable.
- Automation providers like Microsoft and Amazon provide drag-and-drop tools that simplify the process.
8. Leverage Enterprise-Grade APIs
- Enterprise data can be extracted and loaded into data warehouses via secure APIs.
- Aggregate different data sources into a single data warehouse hosted by Amazon, Google, or Microsoft
Pricing Range for Data Warehouse Automation Software?
Many Data Warehouse Automation providers don't publish typical pricing online. From our research, it's reasonable to expect to pay $1,000 to $3,500 per user monthly.
These costs are usually all-inclusive, with data integration connectors, automation scripts, visualization software, ETL functionality, data backups, etc.
Data Warehouse Automation Tools & Providers
WhereScape
WhereScape is a popular data warehouse automation software that enables users to build, deploy, and manage data warehouses quickly and efficiently.
Matillion
Matillion is a cloud-based data integration and ETL software that provides data warehouse automation capabilities for building data warehouses on popular cloud platforms such as Amazon Web Services (AWS), Google Cloud Platform (GCP), and Microsoft Azure.
Informatica
Informatica is a popular data integration and ETL software that provides data warehouse automation capabilities for building data warehouses, data marts, and data lakes.
Snowflake
Snowflake is a cloud-based data warehousing platform that provides automation capabilities for building and managing data warehouses.
Azure Synapse Analytics
Azure Synapse Analytics is a cloud-based data warehousing platform that provides data warehouse automation capabilities for building and managing data warehouses on Microsoft Azure.
Oracle Autonomous Data Warehouse
Oracle Autonomous Data Warehouse is a cloud-based data warehousing platform that provides automation capabilities for building and managing data warehouses on Oracle Cloud.
Functionality & Features Needed for Data Warehouse Automation
When automating your data warehouse, it is essential to focus on various important aspects and features like:
High performance
Adequate database performance is a much-needed quality for data warehouses. The data operations required for executing complex analysis can be highly resource intensive. The data warehouse design and data models should be developed with performance optimization in mind. A data warehouse should be able to deliver fast results even when working with massive amounts of data.
Optimization
You should also look for further optimization opportunities, such as data compression techniques for optimizing storage. Be it an on-premise or cloud-based data warehouse, you pay more for every extra storage byte you need. So, optimization features are a must-have to save up on costs as well as boost performance. Look into the query designs, data duplication techniques, and similar optimization features provided.
Computing
Pay attention to the computing power and resources required to run your preferred data warehouse solutions. Computational requirements impact the overall running costs of maintaining your data warehouse systems. The more efficiently designed your system is, the more cost-effective it will be.
Data Visualizations
Data visualizations are an important aspect of what makes a data warehouse self-serviceable. The right visualization tool can help you save time on performing complex queries and get you faster insights. They help reduce the need for coding and facilitate easier decision-making and information sharing across the relevant stakeholders.
Dashboards
Like data visualizations, dashboards must be designed intuitively. They should have the necessary functionalities to make your data warehouse system more accessible and easier to use.
Business Intelligence (BI) Tools
BI tools help achieve the business requirements that motivate data warehouse development in the first place. BI tools can work on top of a data warehouse to give you accurate and quantifiable insights to make data-based business decisions. Your data warehouse solutions should be compatible with BI tools or easily integrate with BI systems.
Machine Mearning
Most modern analytical models are developed using ML technologies. A data warehouse system that can be readily integrated with ML technologies will be a considerable asset in further improving your analytical and learning models.
Data-Driven
Ensure your data warehouse system can support the data models and structures applicable to your business cases, like the ones extracted from Salesforce or SAP.
Metadata-Driven
Metadata is data about data. It gives information based on a template, such as the transactional history of any piece of data, its location, author details, origin details, format, structure, etc. Metadata-driven data warehouse development is often found to be more efficient and optimized.