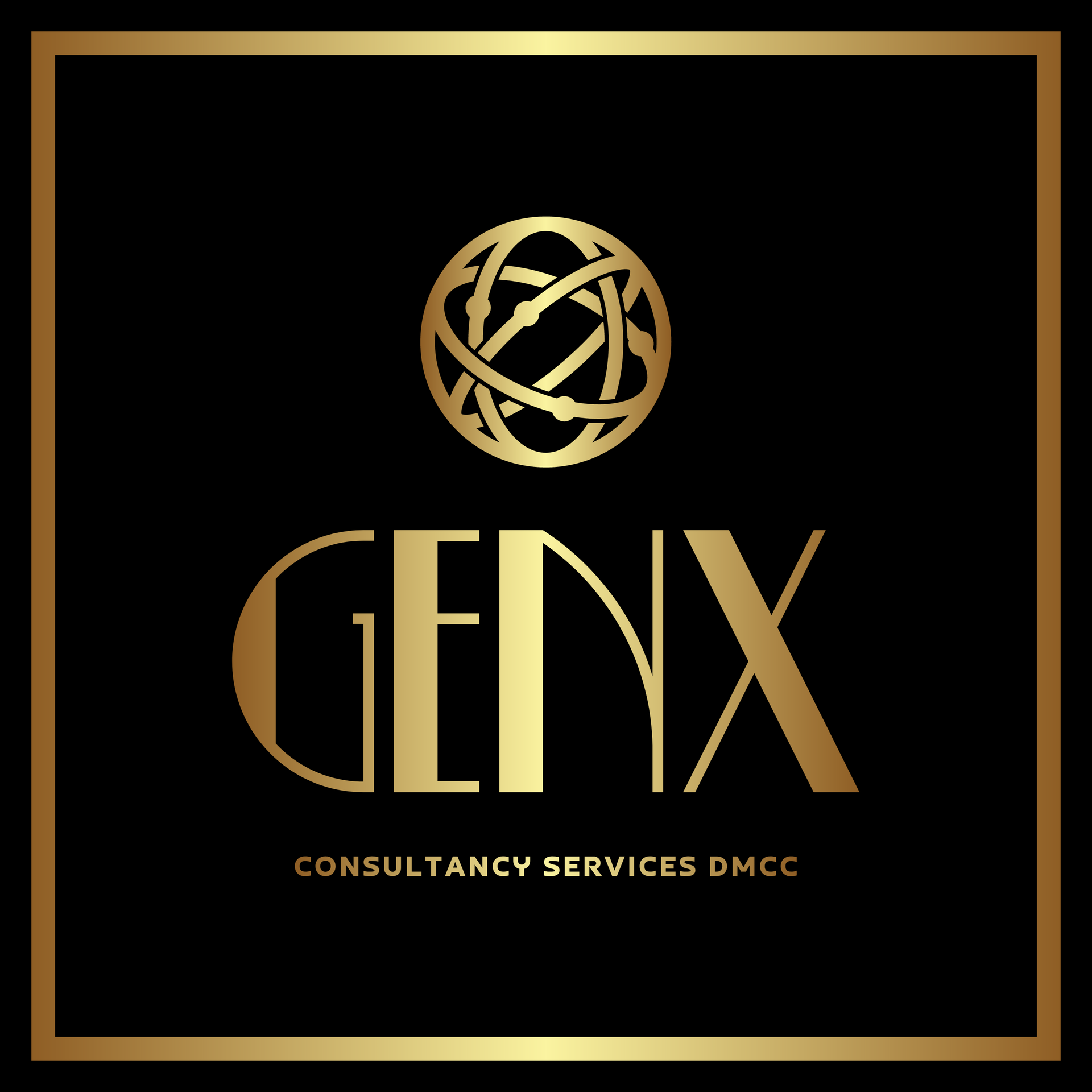
Jeralyn • July 13, 2024
Exploring Dark Data and Its Potential in BI
Potential of Dark Data in Business Intelligence (BI)
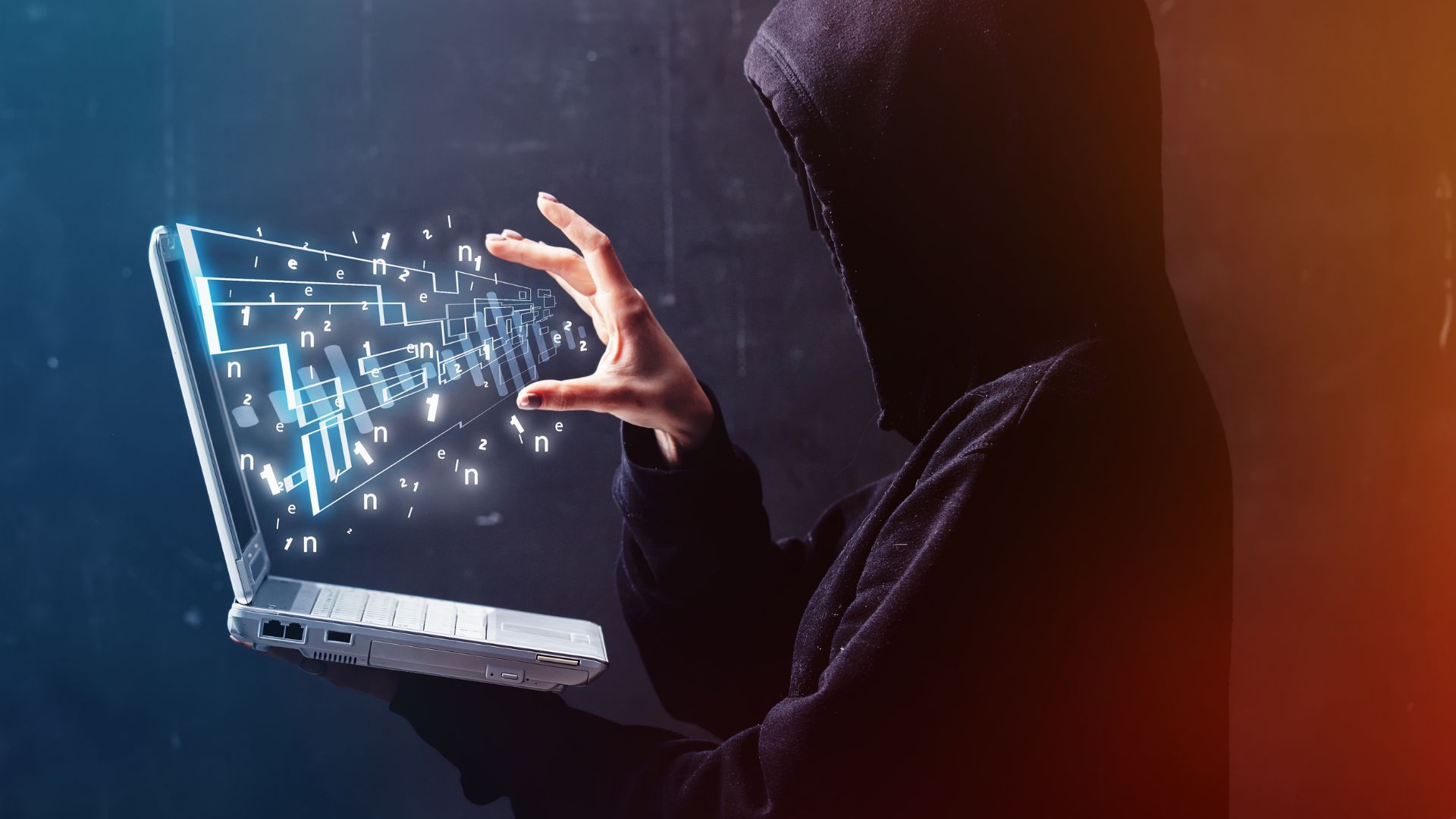
What is Dark Data?
Dark Data refers to the vast amount of unstructured, untapped, and often forgotten data that organizations collect, process, and store during regular business activities but do not use for any meaningful purpose.
Examples include:
- Log Files: Server logs, network logs, and application logs.
- Sensor Data: Data from IoT devices and sensors.
- Customer Interaction Data: Call center transcripts, email communications, and social media interactions.
- Survey Data: Responses from customer surveys that are collected but not analyzed.
- Document Files: Invoices, contracts, and reports stored in digital formats but not systematically used.
Potential of Dark Data in Business Intelligence (BI)
Enhanced Decision-Making
- Comprehensive Insights: Integrating dark data into BI systems can provide a more comprehensive view of the business environment, leading to more informed and effective decision-making.
- Predictive Analytics: Leveraging dark data can enhance predictive analytics models, allowing businesses to forecast trends more accurately.
Customer Insights and Personalization
- Customer Behavior: Analyzing customer interaction data can reveal patterns in customer behavior, preferences, and sentiments, enabling personalized marketing strategies.
- Improved Customer Service: Understanding customer issues and feedback from unstructured data like call center logs can help improve service quality and customer satisfaction.
Operational Efficiency
- Process Optimization: Examining operational logs can identify inefficiencies and areas for process improvement.
- Proactive Maintenance: Analyzing sensor data from machinery can predict maintenance needs, reducing downtime and maintenance costs.
Risk Management
- Fraud Detection: Analyzing transaction logs and other related dark data can help identify fraudulent activities.
- Compliance: Ensuring that unstructured data is reviewed can help organizations stay compliant with regulations by identifying and mitigating risks associated with data breaches or non-compliance.
Innovation and Development
- Product Development: Customer feedback and interaction data can provide insights into product performance and areas for improvement.
- Market Trends: Identifying emerging trends through the analysis of social media and other external data sources can drive innovation.
Challenges in Leveraging Dark Data
Data Quality and Integration
- Unstructured Nature: Dark data is often unstructured and stored in various formats, making it challenging to integrate into BI systems.
- Quality Issues: Ensuring data quality, consistency, and accuracy is critical when incorporating dark data into analytics.
Storage and Management
- Volume: The sheer volume of dark data can be overwhelming, requiring significant storage and computational resources.
- Management: Efficient data management practices are needed to handle and organize dark data effectively.
Privacy and Security
- Sensitive Information: Dark data may contain sensitive information, posing privacy and security risks if not handled properly.
- Regulatory Compliance: Organizations must ensure that their use of dark data complies with data protection regulations such as GDPR and CCPA.
Analytical Complexity
- Advanced Tools: Leveraging dark data often requires advanced analytical tools and techniques such as natural language processing (NLP) and machine learning.
- Skilled Personnel: There is a need for skilled data scientists and analysts capable of extracting insights from complex and unstructured data sets.