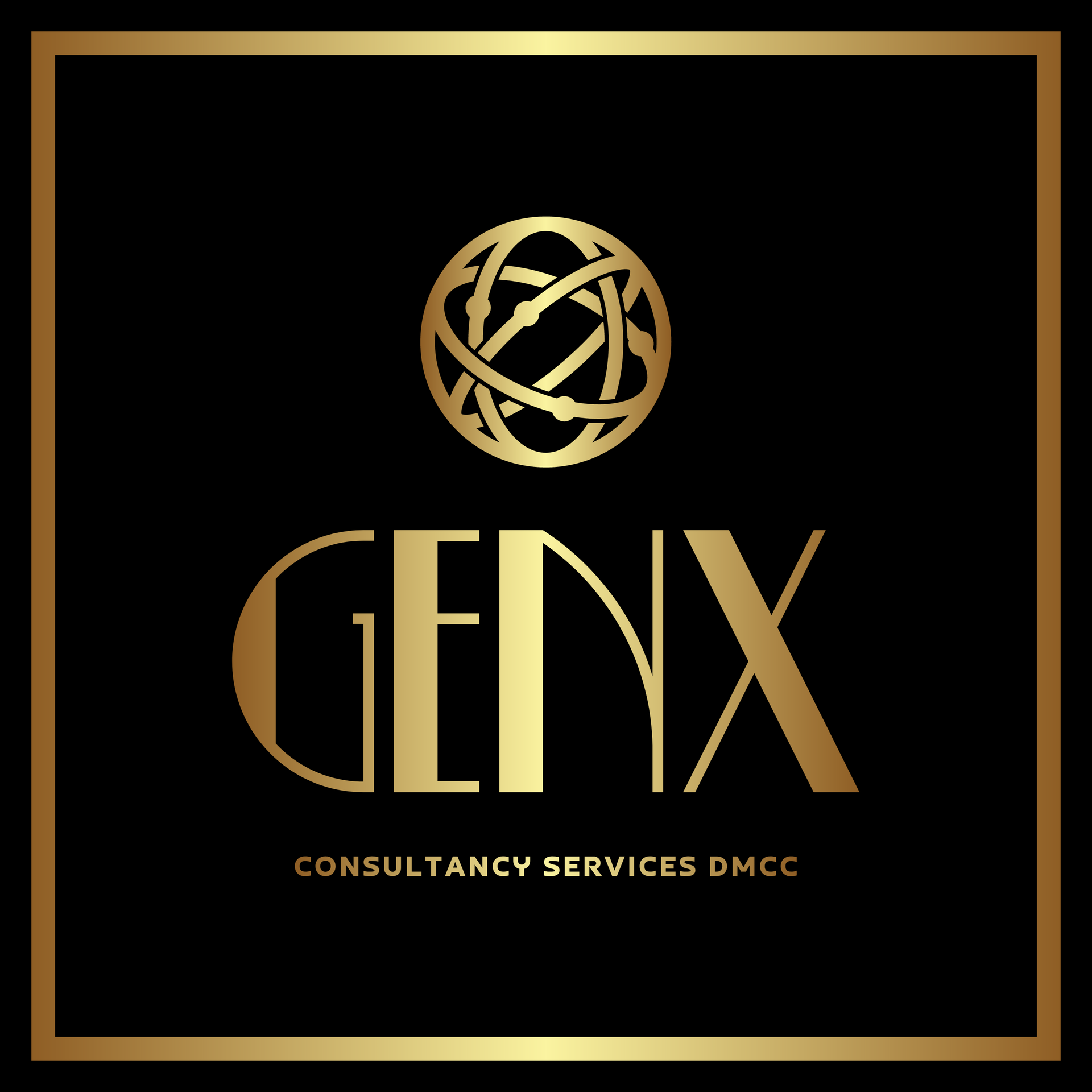
Data Integration Use Cases
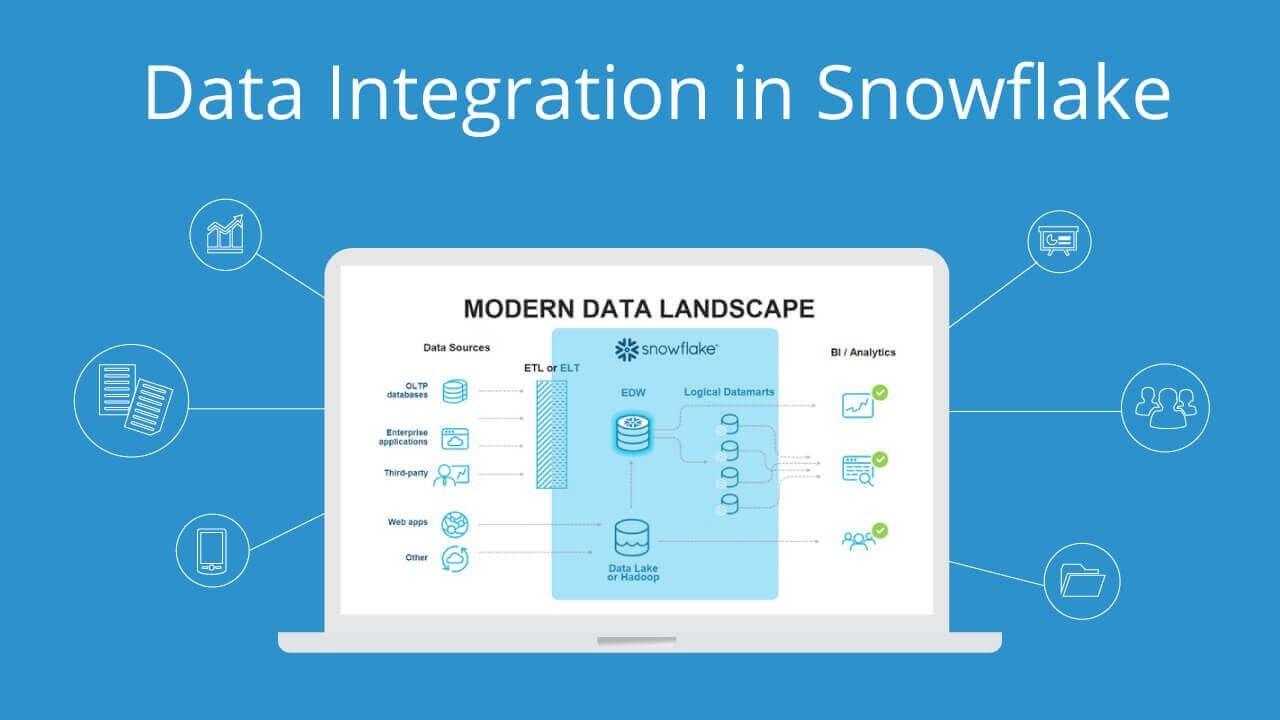
Integrating Data with Snowflake Data Cloud
Snowflake is a cloud data warehouse service usually available on a pay-as-you-go SaaS model.
- Snowflake's architecture separates the application's storage and computing resources.
- It can be used with all major cloud platforms, such as Microsoft Azure, AWS, and GCP.
- Many data science teams use Snowflake for data integration from multiple sources.
- You can use its 'storage' and 'compute' parts independently.
- Snowflake can be readily used alongside your existing ETL solutions and help with efficient real-time data operations via its quick sharing features. It is a perfect solution for organizations requiring a flexible data integration solution.
Data Integration Use Cases
A Snowflake data integration solution would benefit most data integration use cases, like:
Analytics pipeline enhancement
- Moving from batch loads to real-time data streams can significantly enhance the performance of your analytics applications. And this can be made possible with the help of Snowflake data integration.
- Snowflake data integration helps provide a uniform, secure, concurrent data warehouse across your organization. This, in turn, enhances your analytical applications as you can always access consistent and latest data.
Persistent query results
- Snowflake makes use of caches to deliver faster results. When nothing has changed with your data source, it shouldn't take more time to get the same type of reports repeatedly.
- With the Snowflake data service, you can fetch the latest query results from the cache and get your reports faster.
Breaking down data silos
You run into several issues when your data systems are isolated into silos across your departments. You could face redundancy, inconsistent data, lowered performance and decreased data quality.
Snowflake can avoid these problems and help you avoid silos. It can thus lower your operational costs, increase your performance and drive better data-based decision-making.
Improving business intelligence
With Snowflake, you can easily integrate customer data from multiple channels and use it to understand user behavior and yield actionable insights. You can use the information thus gained to improve your product further and enhance customer satisfaction.
Implement effective data exchange
Snowflake is the best tool for establishing a data exchange, where you can communicate in real time with collected data.
It helps you create data exchange and share data across your departments, clients, and partners as and when required.
Snowflake ETL Examples
You would extract data from multiple data sources, transform it into a more efficient structure, and load it into the final target system. The target system could be any data warehouse, cloud storage, or application. Historically, engineers had to write Python scripts for automation and validation. The ETL process provides the framework for collecting and integrating data from multiple sources into a unified data warehouse.
Snowflake supports ETL and can be used with various data integration tools. It also supports a similar process called ELT, where the loading step is carried out before the data transformation step.
Some of the unique features of Snowflake integrations are:
- COPY command: To perform bulk loading operations.
- Supports numerous data types: Numeric, string, date-time, structured and semi-structured, arrays, geospatial data, blobs, and more
- Stored procedures: These combine SQL and Javascript and can be used to implement logical procedures for data operations.
- Streams: Help you keep track of any data updates or changes
- Tasks: These can be set up and scheduled to carry out data operations
- Snowpipes: Allow for continuous loading of data in the form of micro-batches.
Snowflake Data Integration Best Practices
Data extraction
- The data extraction stage is where data is gathered from one or more data sources. It can also be called data ingestion. The sources can be anything from a website, database, web data, documents, spreadsheets, app data, and more.
- An excellent way to optimize your data ingestion with Snowflake is to use native methods such as Snowpipe.
- Snowpipe is a data ingestion tool that allows you to load data as soon as it reaches the staging stage. It helps make optimal usage of your available resources.
- You can choose a real-time streaming or batch-loading method to extract data with Snowflake.
- Real-time streaming is best suited when you want immediate insights and quick decision-making.
Data transformation
- Data can come in multiple formats when ingested from various data sources. Data might also demand differing computation and storage requirements.
- Here are tips that you can use to optimize the transformation process:
- Retain the raw data history to build automatic schemas. This could also help with ML algorithms and data analytics in the future.
- Use multiple data models as it gives you better results when reloading and reprocessing data.
- Use the right tools for suitable file formats. Snowflake provides native modules to handle a wide range of file formats. Do read up and use them appropriately.
- Develop a plan and transform data in a step-by-step manner.
Data loading
- The data loading stage involves data migration, loading the data into the snowflake data warehouse, and using the best data platform. The best-recommended way to load data into Snowflake is to use the COPY command.
- Get a good understanding of this command to be able to make optimized loading of data.
- Before loading, stage data in possible locations such as an AWS S3 bucket, Azure container, or an external location.
- A batch load is better for running repetitive procedures on data such as daily reports. You can also alternate between these methods or use them as and when necessary.
Data governance
The data governance stage refers to managing data workloads and the compliance that must be handled while working with the data.
Here are some tips for effective data governance:
- Identify the data domains you use and define your data control points. Make sure your automation and workflow processes are well-defined.
- Establish and document the scope of data, such as personally identifiable data (PII), often found with CRM software like Salesforce.
- Identify repetitive processes, automate them, and review them periodically to further improve your data governance structure.