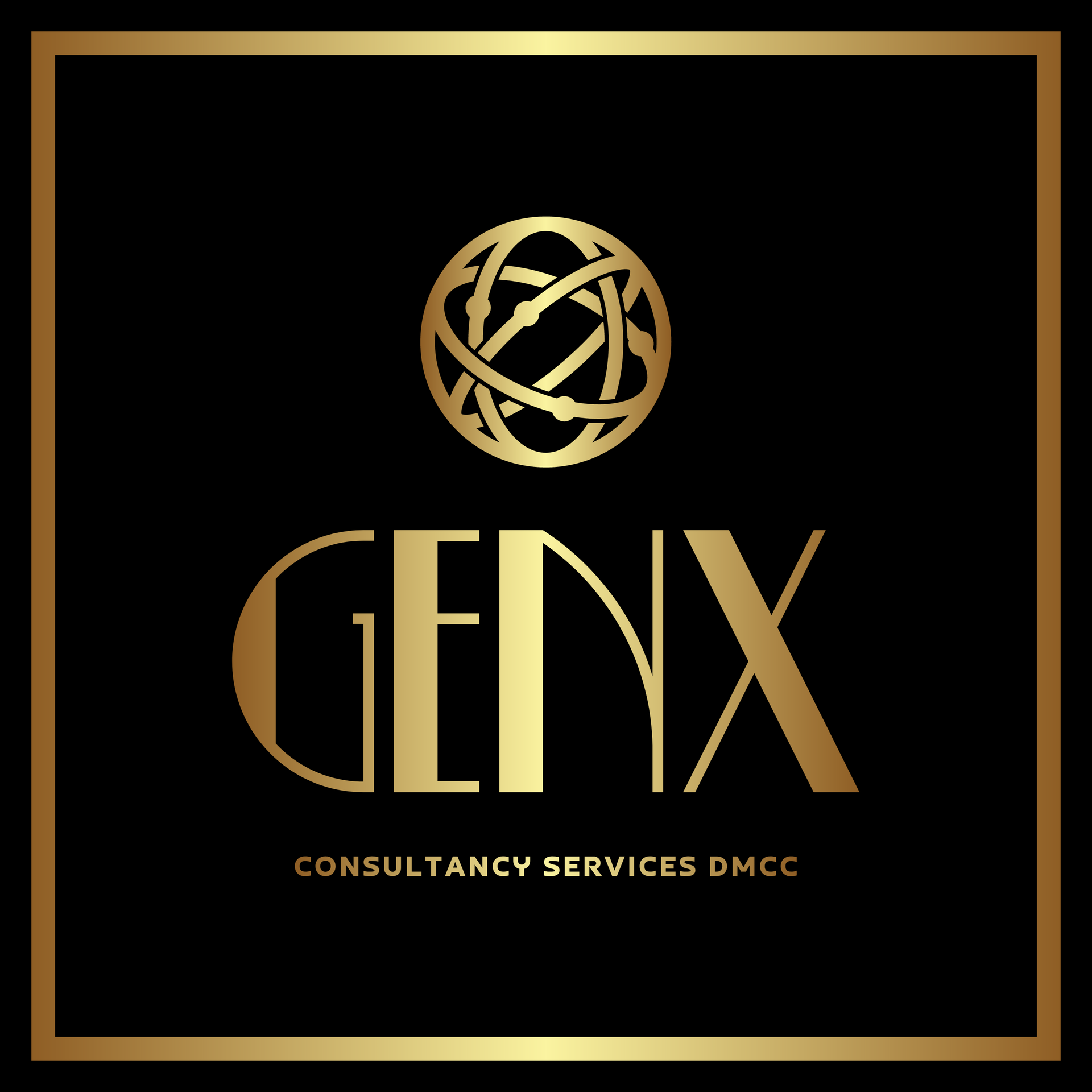
BigQuery Benefits with ELT
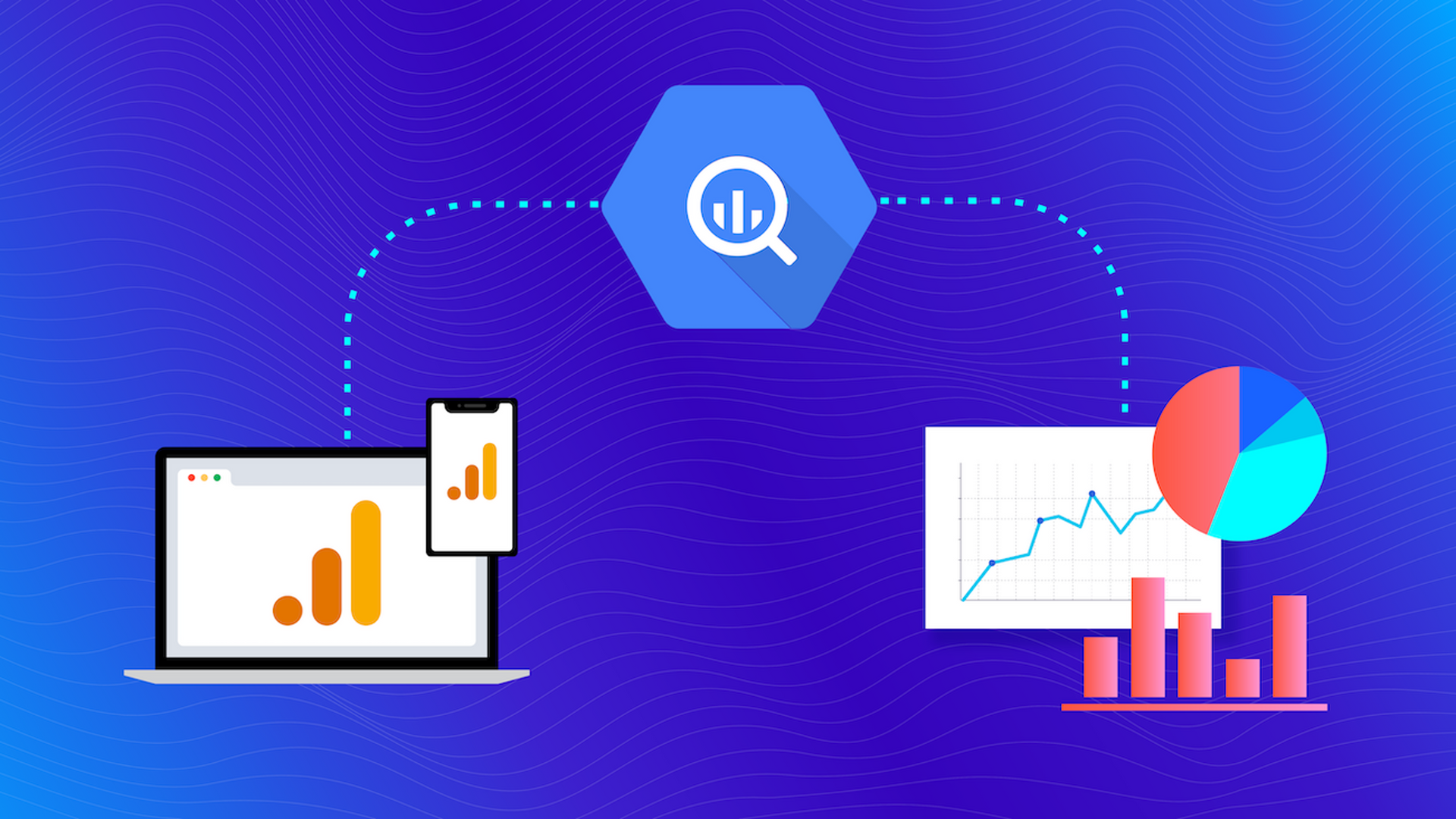
BigQuery Benefits with ELT
Excellent performance and speed
- BigQuery uses distributed serverless computing model and various replication techniques to process large datasets and perform complex queries quickly.
- The query execution engine used by BigQuery is called Dremel and is exceptional when scaling up on demand.
- Dremel can process data rows in the count of trillions within mere seconds.
- BigQuery also employs advanced systems like the Colossus distributed file system and the Juniper Network connecting the Colossus storage and Dremel, all designed for excellent performance optimization and seamless scalability.
Ease of use
- Google BigQuery allows you to run queries like the standard SQL.
- It has an easy-to-use interface. You need not have expensive hardware or extensive coding knowledge to work with BigQuery
Fast real-time analytics
With all the performance-enhancing features provided by BigQuery, running powerful analytical applications becomes much easier. You get quick insights from real-time data through sleek dashboard visualization.
Flexible pricing models
- The common pricing model in BigQuery is pay-per-use, which allows you to pay only for the resources used.
- It also provides a flat pay alternative, where you pay a flat rate depending on the resource range you would be using.
- This flat pay model is best suited for companies with a fixed storage size and a predictable estimate of the number of queries and operations they would need to run on the data warehouse.
Fine-grained access control
- BigQuery allows you to define a fine level of access control starting from field level to project level.
- This helps you stay compliant with all the data standards and regulations put forth by regulatory bodies like HIPAA and GDPR.
Integration with the wider Google Cloud Services
- Google has a wide range of productivity tools and internet services, which can all be easily integrated into your BigQuery data warehouse solution.
- All your data from Google Sheets, email, data studio, and so on can be seamlessly integrated with BigQuery with no extra effort.
Building an ELT data pipeline for BigQuery
The basic process of creating an ELT pipeline applies to BigQuery as well. You identify your data sources, set up the connectors, and build the Extract and Load operations which store the data in your BigQuery data warehouse.
- ELT stands for Extract, Load, and Transform, which is exactly what an ELT data pipeline executes in a sequence.
- Data is extracted from its source and loaded onto the target, the BigQuery data warehouse in this case.
- Then depending on the requirements, the loaded data can be transformed to be compatible with the data analytical apps and BI tools (business intelligence tools) that run on top of the data.
- There are many reasons why an ELT data pipeline serves a cloud-based data warehouse better compared to the traditional ETL method and ETL tools.
- ELT lets you take full advantage of the cloud-native environment. Thus, you can avoid the costly and time-consuming transformation processes on the client side and move/replicate the data to the cloud data store.
- ELT data pipelines can be easily automated, making them an efficient option for repetitive tasks and scheduled data integration tasks. This helps large organizations maintain an up-to-date and accurate data management system.
Best practices for ELT with BigQuery
You don't have to perform any transformation in the ELT pipelines, so you can directly load the data to BigQuery using the Data Transfer Service. The GCS is often used as a staging platform for ETL pipelines to easily transform data before loading.
Try to compress your data before loading to improve data transfer speed. Here are some pointers to help with data compression:
- Use Avro binary format whenever possible, as it is the most efficient format for loading data.
- Other efficient formats include Parquet and ORC format
- If your data format is already in CSV or JSON files, you can load them directly, as these formats load faster when they are uncompressed.
- Make use of streaming inserts to load data without any delays. You can use SDKs and services like Data flow to perform stream inserts.
ELT Tools for BigQuery
- ELT tools are essential to set up efficient ELT data pipelines. Without these tools, setting up the various data connectors, staging, loading, and transformation tasks can be quite cumbersome.
- These tools can greatly reduce the time spent on coding and can help your data engineers focus on creating efficient data management systems that provide value in a quick turnaround time.
- The basic purpose of an ELT approach tool is to replicate data from multiple data sources and feed it to your centralized repository, which could be a data lake/cloud data warehouse solution.
- They also help you automate the data pipelines, minimize errors, and ensure your data quality is maintained throughout the process.
- ELT tools also offer the advantage of ensuring your data complies with data standards and regulations.