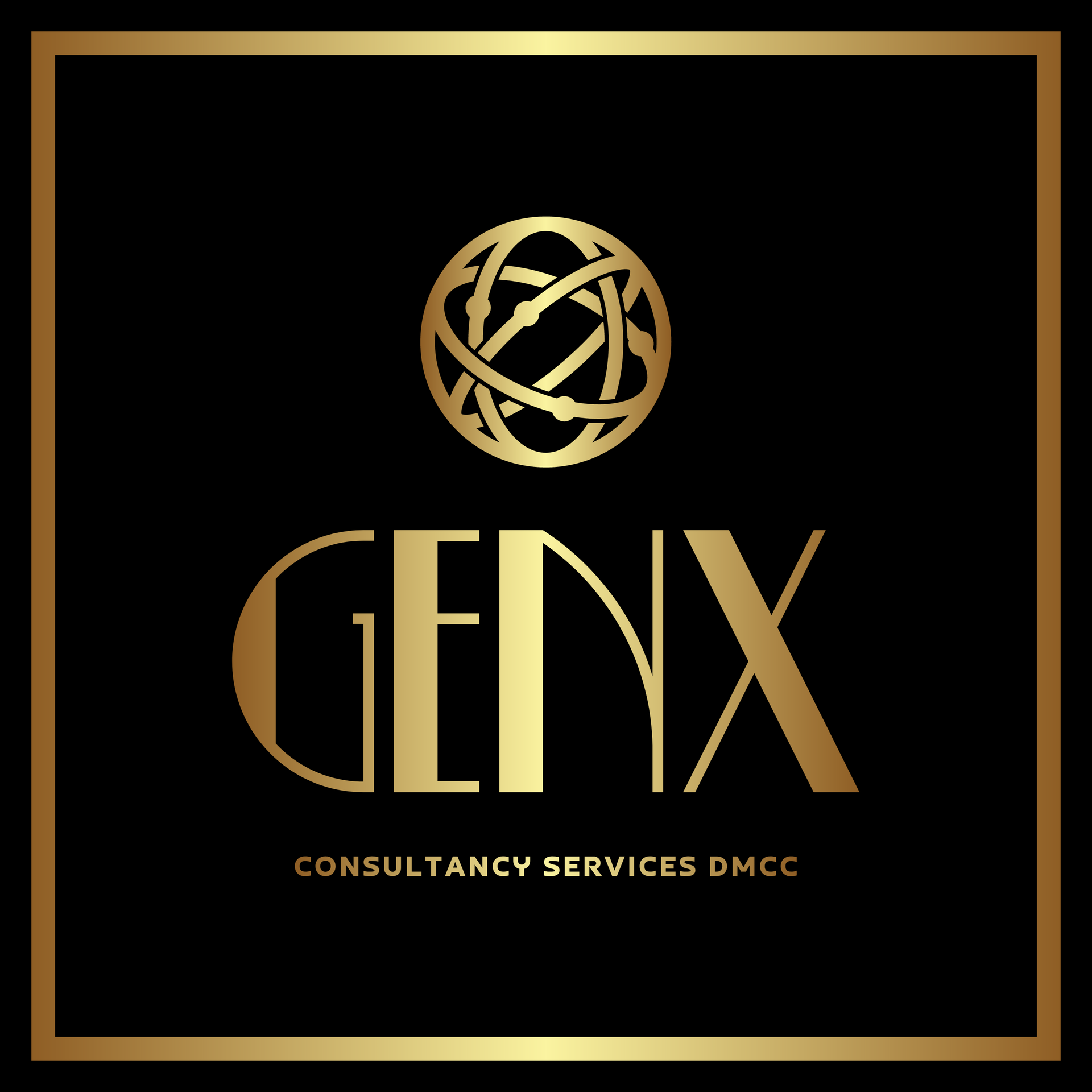
What Hadoop is used for?
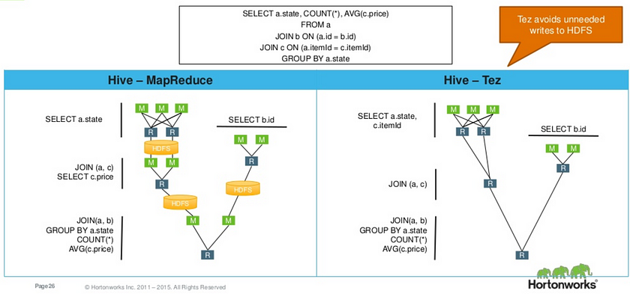
Hadoop is an open-source software framework used for distributed storage and processing of large datasets on clusters of commodity hardware. It was created by the Apache Software Foundation and is widely used in big data applications.
The core of Hadoop is the Hadoop Distributed File System (HDFS), which provides a fault-tolerant and scalable way to store large amounts of data across multiple machines. Hadoop also includes a programming model called MapReduce, which allows developers to write parallel processing algorithms that can be executed across the nodes of a Hadoop cluster.
It has three main points:
- A software platform. It runs on cluster of nodes (servers).
- A distributed storage. It’s a filesystem that stores files across multiple hard drives. Though, we can visualize all of them as a single file system. It keeps copies of the data and recover automatically.
- A distributed processing. All processing (like aggregation of data) is distributed across all nodes in parallel.
Hadoop was developed at Yahoo! to support distribution for the “Nutch”; a search engine project. While the data storage is inspired by Google File System (GFS), and data processing is inspired by MapReduce.
What Hadoop is used for?
It’s for batch processing, but some solutions built on top of Hadoop provide interactive querying (CRUD applications), and we’ll go through them.
Batch processing means processing of batch of separate jobs (tasks) at the background given the fact it wasn’t triggered by the user (no user interaction), such as calculating user monthly bill.
The scaling and distribution of storage and work, is horizontal.
With vertical scaling, still, we’ll face issues like: disk seek times (one big disk vs many smaller disks), handle hardware failures (one machine vs data copied among different machines), processing times (one machine vs in parallel).
Hadoop is used in many different industries for a variety of purposes, such as:
- Business intelligence: Hadoop can be used to store and process large amounts of data from various sources, enabling businesses to perform advanced analytics and gain insights into their operations, customers, and markets.
- Fraud detection: Hadoop can be used to analyze large volumes of data to identify patterns and anomalies that may indicate fraudulent activity.
- Healthcare: Hadoop can be used to store and analyze patient data, medical images, and other healthcare-related information to improve patient outcomes and streamline healthcare operations.
- E-commerce: Hadoop can be used to analyze customer data, such as purchase history and clickstream data, to improve customer experience, personalize recommendations, and optimize pricing and promotions.
- Social media: Hadoop can be used to analyze social media data, such as tweets and posts, to gain insights into customer sentiment, brand reputation, and market trends.
Overall, Hadoop is a versatile tool that can be used in many different applications where large amounts of data need to be processed and analyzed.
Core Hadoop
— What’s part of Hadoop itself?
HDFS, YARN, and MapReduce. These solutions are built on top of Hadoop directly. Everything else is integrated with it.
HDFS
It’s a file system. The data storage of Hadoop. Just like Amazon S3 and Google Storage.
HDFS can handle large files that are broken and distributed across machines in the cluster. It does this by breaking the files into blocks. And so, no more huge number of small files.
We can access the distributed data in parallel (say, for processing). It stores a copies of a block on different machines. So, machines talk to each other for replication.
First. A name node that tracks of where each block lives, and data nodes that have the blocks.
When reading …
- Our application uses a client library that talks to name node, and it asks for a specific data (file).
- The name node replies back with the data node(s) that have the data.
- The client library talks to these data nodes, and sends the result back to us.
When writing …
- The client library will inform the name node about the writing operation.
- The name node will reply back by where to store this file, and new entry for that file has been created.
- The client library go and save the file data in the given location (node X).
- The node X then talks to other machines for replication. And send back to the client library acknowledging the data has been stored and replicated.
- The client library forwards that acknowledgment to the name node that data has been stored, and where it was replicated.
- The name node updates its tracking metadata.
The name node is obviously a single point of failure. However, It’s important to have a single node as they may not agree on where data should be stored. To solve the problem, a backup name node can take over when the main node fails.
YARN
YARN: Yet, another resource negotiator. The resource (CPU, memory, disk, network) management layer of Hadoop.
It splits the work — in this case resource management and job scheduling and monitoring, across the nodes, taking care data ‘processing’.
It was part of Hadoop, but then split out, allowed data processing tools like graph processing, interactive processing, stream processing, and batch processing to run and process the stored data. We’ll see examples next.
- The client talks to YARN, and YARN then spin up a node running application master.
- YARN allocates resources to the applications running, and passes the jobs coming to the master to be executed.
- The application master talk to other (worker) nodes, which are running containers that execute the job, and talk to HDFS to get the data to be processed and save the result back.
- The application master can also ask for more resources from YARN, track their status and monitor the progress.
- Each node has its own node manager, which tracks what each node is doing, and constantly report back to YARN with the node status.
Mesos
It’s an alternative to YARN, yet it’s still different. It’s used by Twitter, not directly associated with Hadoop, and has a more broad system.
— Mesos Vs YARN
- Mesos manages the resources across the data centers, instead of just Hadoop. Not only about the data but also web servers, CPU, etc.
- YARN, you give it a job, and it figures out how to process it. Mesos, you give it a job, and replies back with the available resources, and then we decide whether to accept or reject.
- YARN is optimized for long, analytical jobs, while Mesos is more general purpose; can be for short, or long jobs.
— When to use Mesos?
To manage and run on top of your cluster, not only Hadoop. We can use both YARN to manage nodes for Hadoop, while Mesos manages nodes for web servers, and everything else.
MapReduce
It’s the data processing of Hadoop. It consists of Map: run a function on nodes in parallel, and Reduce: aggregate the results of all nodes.
It distributes the processing by dividing among partitions (nodes). A mapper function will be applied to each partition, and a reducer function to aggregate results of mapper.
Let’s take an example. The task here is to get the count for every word across massive amount of text data. A MapReduce job is composed of three steps:
- Mapper. Given text data, for each input, transform it to key-value pair. In this case, the key is the word, and value is just 1 (will be aggregated).
- Shuffle & Sort. MapReduce automatically groups same keys, and sort them. This step happens right before every reduce function. So, we’ll end up having the word as a key, and the value is an array of 1s.
- Reduce. Given a list of all the words, aggregate. So, for every key (word), we’ll get the count (value=[1,1,1,…]). The reducer gets called for each unique key.
— How MapReduce distributes the processing?
Remember YARN?. If so, then this should be familiar since MapReduce runs on top of it.
So. It talks to YARN saying, “Hey, I want to process this job”. And the same steps explained above will kick off. The only difference here is containers (workers) are running map/reduce jobs.
— Failures?
The application master restarts the worker (preferably on a different machine) when a worker fails. YARN will restart the application master if itself failed.
Tez
It runs on top of YARN. It’s an alternative to MapReduce. In fact, we can configure some of the tools that run on MapReduce by default like Hive and Pig to run on Tez instead. Tez tries to analyze the relationship between the steps & independent paths, and figure out the most optimal path for executing the queries.
It tries to run the steps in parallel instead of in sequence as in MapReduce, as It has a complete view or awareness of the job, before running it. It also optimize the resource usage, and minimize the move of data around.
This is called “Directed Acyclic Graph (DAG)”. It about accelerating the jobs.
Spark
It can set on top of YARN, and an alternative to MapReduce, allowing to use programming language like Python, Java or Scala to write queries. It uses the idea of map/reduce, but not the exact implementation.
Besides, It provides many functionalities for ML, data mining, streaming. And, uses DAG, same as in Tez.
— How It works?
It’s based on RDD; An object represents the dataset thats actually being distributed across nodes in the cluster and has a set of built-in methods.
So, we, just deal with a dataset; a single object, though, it’s distributed.
Hadoop has become a popular tool for processing large datasets in many industries, including finance, healthcare, retail, and telecommunications. It is often used in conjunction with other big data technologies such as Apache Spark and Apache Hive.