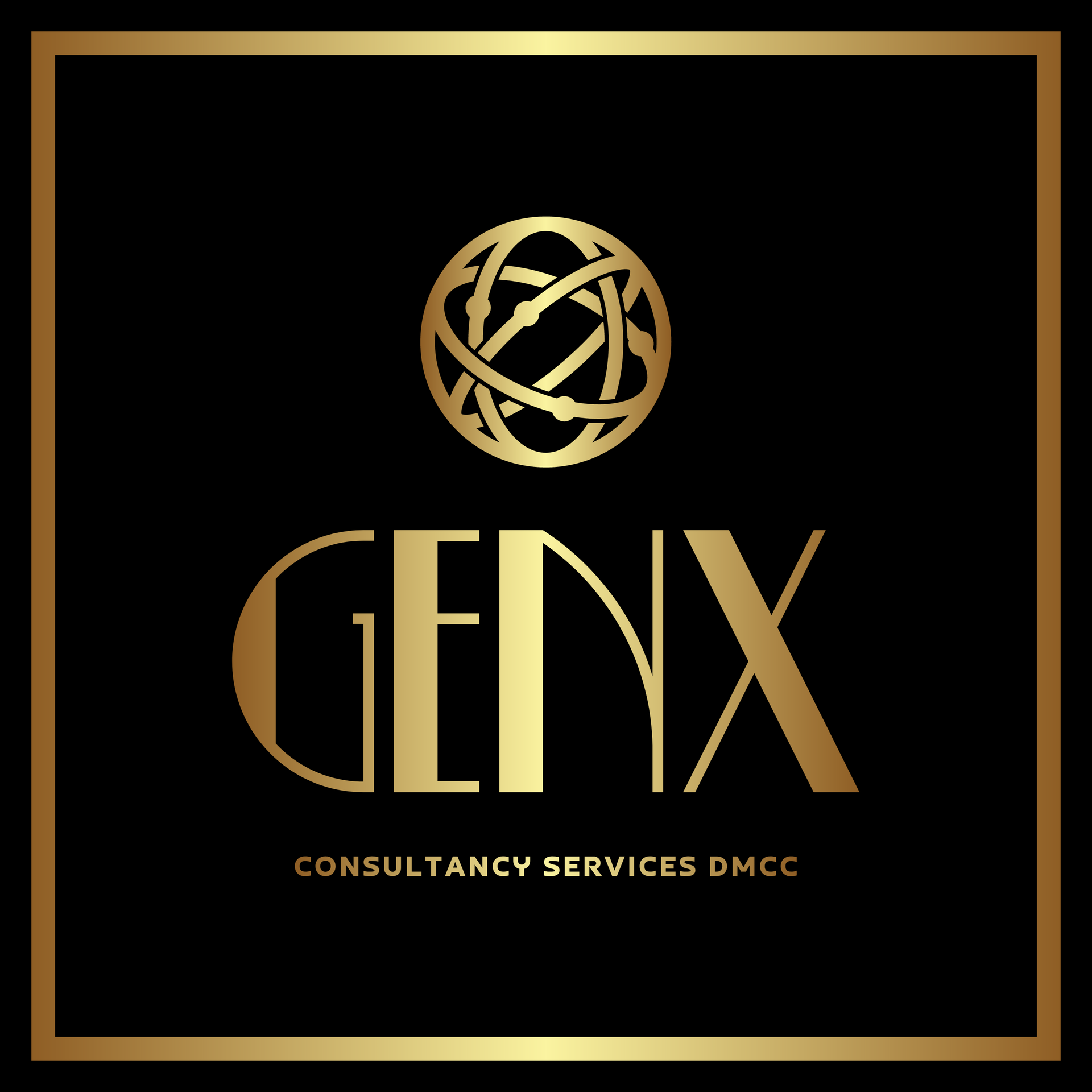
Microsoft Fabric vs Databricks: Use Cases
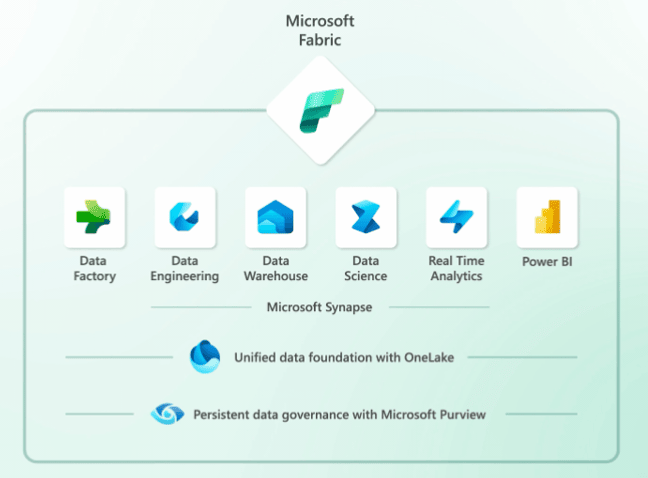
What is Microsoft Fabric?
Microsoft Fabric is an all-in-one analytics platform launched in May 2023. It provides a unified environment for data engineering, data science, machine learning, and business intelligence.
Fabric is built on top of Azure Synapse Analytics and Azure Data Factory. It includes a variety of other services, from Azure Data Fabric architecture e.g. Power BI, Azure Databricks, and Azure Machine Learning.
What is Databricks?
Databricks is a unified analytics platform, built on top of Apache Spark. It provides a variety of features for data processing, data warehousing, and machine learning. It was founded in 2013.
Databricks is a cloud-based platform and is available on all major cloud providers, including AWS, Azure, and Google Cloud Platform.
Its comprehensive set of features, from optimized Spark performance to collaborative workspaces, makes it an invaluable tool.
Microsoft Fabric vs Databricks: Architecture and Components
Microsoft Fabric’s Unified Data Platform
Microsoft Fabric stands on the foundation of a thoughtfully crafted architecture, integrating a spectrum of essential components to address varied data requirements – a fully managed data management platform that aims to unite multiple roles within an organization.
It consists of the following capabilities:
- Data Lake: Serving as the cornerstone, the Data Lake is a robust and expandable storage facility. It’s proficient in housing diverse data types, whether structured or unstructured, guaranteeing data consistency and easy access.
- Data Engineering: This component is the backbone of the architecture, focusing on the transformation and optimization of data. It ensures data is not just purified but also ready for in-depth analysis.
- Data Integration: Bridging the gap, the Data Integration platforms seamlessly merge various data sources. They guarantee smooth data movement and synchronization across different platforms, simplifying data amalgamation.
- Machine Learning: For enthusiasts eager to tap into AI’s potential, the Machine Learning platform is invaluable. It’s designed to streamline the development, fine-tuning, and rollout of sophisticated machine learning algorithms, propelling automation and foresight.
- Business Intelligence: The Business Intelligence tool specializes in morphing raw data into actionable intelligence. It boasts advanced visualization tools, enabling users to probe data deeply and garner crucial insights, facilitating data-driven decisions.
Databricks’ Lakehouse Data Framework
Databricks champions the Lakehouse design, an elegant fusion of data lakes’ and data warehouses’ primary features.
This design is anchored around key components:
- Data Sharing: Databricks advocates for transparent data sharing, fostering smooth collaboration across different platforms. This ensures datasets, models, dashboards, and notebooks are shareable while maintaining rigorous security and governance protocols.
- Data Management and Engineering: The platform optimizes data intake and handling procedures. Leveraging automated ETL and the agility of Delta Lake, Databricks metamorphoses your data lake into a central hub for all data forms.
- Data Warehousing: Databricks guarantees users access to the most up-to-date and holistic data. Harnessing Databricks SQL, it delivers unmatched price-to-performance metrics compared to conventional cloud data warehouses, facilitating swift insight generation.
- Data Science and Machine Learning: The Lakehouse is the cornerstone for Databricks Machine Learning. It’s an all-encompassing solution addressing the entire machine learning spectrum. From data preparation to deployment, the platform, enriched with top-tier data pipelines, expedites machine learning endeavors and enhances team efficiency.
Microsoft Fabric vs Databricks: Use Cases
Microsoft Fabric Use Cases and Key Features
Fabric bundles together different Azure technologies on top of its OneLake system and bundles it all up with additional features such as Microsoft’s AI assistant, CoPilot, and a host of other technologies that aim to increase productivity and awareness within different teams.
- Microservices Architecture: Microsoft Fabric is designed from the ground up to support microservices patterns. This architecture allows developers to build applications as small, independent services that can be developed, and scaled individually.
- Container Orchestration: With the rise of containerization, Azure Data Fabric architecture provides built-in support for orchestrating containers. The feature allows developers to deploy and manage both Windows and Linux containers.
- Stateful Services: Unlike some other platforms that only support stateless services, Microsoft Fabric architecture supports stateful services. This means that the platform canmaintaine user sessions or events without relying on external databases or caches.
- Scalability and Load Balancing: The platform is designed to handle large-scale applications. It can automatically balance loads, ensuring that each service instance gets its fair share of requests. As demand grows, Microsoft Fabric can scale out the necessary services to meet the increased load.
- Rolling Upgrades and Rollbacks: Deploying updates and new features is a breeze with Microsoft Fabric architecture. It supports rolling upgrades, meaning that new versions of a service can be deployed without downtime. If something goes wrong, it also supports automatic rollbacks to the previous stable version.
Databricks Use Cases and Key Features
Databricks’ architecture consists of various platforms and integrations that work together to provide a unified workspace. Here they are, along with the benefits:
- Unified Analytics Platform: Databricks brings together big data and AI in a single platform. Thus, it eliminates the need for disparate tools. This unified approach accelerates innovation by allowing data teams to collaborate more effectively.
- Apache Spark Integration: As the brainchild of Apache Spark developers, Databricks offers optimized Spark performance. Users can run large-scale data processing tasks with faster speeds and improved reliability compared to standard Spark deployments.
- Interactive Workspaces: Databricks provides collaborative, interactive notebooks. These support multiple programming languages, including Python, Scala, SQL, and R. The notebooks facilitate collaborative data exploration, visualization, and sharing of insights.
- MLflow Integration: Databricks has integrated MLflow, an open-source platform for managing the machine learning lifecycle. This allows data scientists to track experiments, package code into reproducible runs, and share and deploy models with ease.
- Delta Lake: One of Databricks’ standout features is Delta Lake. This is a storage layer that brings ACID transactions to Apache Spark and big data workloads. It ensures data reliability, improves performance, and simplifies data pipeline architectures.