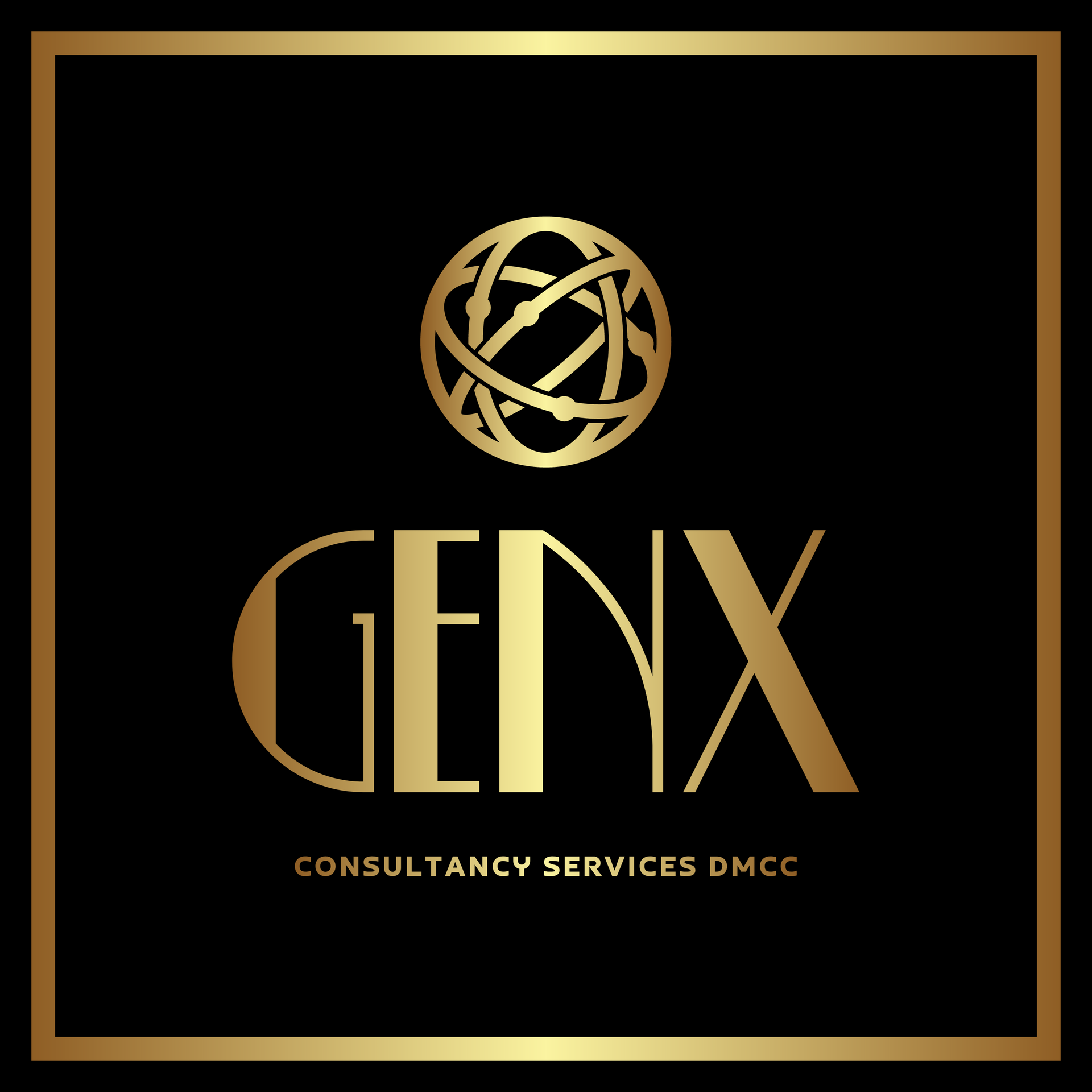
The Need for Explainable AI in Business Intelligence
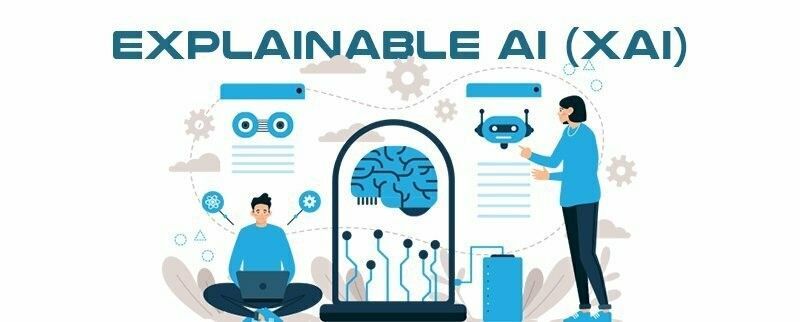
In the present day, artificial intelligence (AI) has seamlessly integrated into our daily lives, transforming numerous industries with its remarkable capabilities.
Among these, the domain of business intelligence has seen substantial advancements owing to AI. Notably, the emergence of Explainable AI (XAI) has empowered businesses to leverage AI technologies while comprehending the rationale behind the decisions made by these sophisticated systems. This article will delve into the ascendancy of Explainable AI within the realm of business intelligence, elucidating its significance and potential implications for organizations.
Understanding Explainable AI
Explainable AI, as the name suggests, refers to the AI systems that can provide understandable explanations for their decisions or predictions. Traditional AI models, such as deep learning neural networks, operate as black boxes, making it difficult for humans to comprehend the underlying reasoning behind their outputs. This lack of transparency has limited the adoption of AI in critical domains where trust and interpretability are paramount. Explainable AI aims to bridge this gap by incorporating transparency and interpretability into AI systems.
The Need for Explainable AI in Business Intelligence
In recent years, businesses have increasingly relied on
AI
and
machine learning (ML) algorithms to analyze vast amounts of data and extract meaningful insights. This has enabled organizations to optimize their operations, enhance customer experiences, and drive innovation. However, the adoption of AI in sectors such as finance, healthcare, and legal services has faced significant challenges due to the lack of transparency and interpretability in traditional AI models.
Consider a scenario in the financial industry where an AI system is responsible for approving or rejecting loan applications. Without explainability, the decision-making process becomes opaque, leaving loan applicants clueless about the reasons behind the rejection. This lack of transparency not only hampers customer trust but also raises concerns about potential biases present in the AI system. Explainable AI can address these concerns by providing clear and understandable explanations for loan rejections, thereby fostering transparency and mitigating the risk of bias.
Benefits of Explainable AI in Business Intelligence
- Transparency: Explainable AI provides transparency into the decision-making process of AI systems, enabling businesses to understand why certain decisions are made. This transparency helps build trust with customers, regulators, and stakeholders.
- Interpretability: By offering explanations in a language that humans can understand, Explainable AI makes it easier for business users to interpret and act upon the insights provided by AI systems. This empowers organizations to make data-driven decisions with confidence.
- Risk Assessment: With explanations provided by Explainable AI, businesses can assess the risks associated with AI-driven outcomes. Understanding the factors influencing a decision allows organizations to identify potential biases, ensure compliance with regulations, and manage any associated risks effectively.
- Regulatory Compliance: In sectors heavily regulated by compliance frameworks, Explainable AI plays a crucial role. By providing transparent explanations, organizations can demonstrate compliance with regulatory requirements, ensuring fairness and accountability in their AI systems.
- Improved Customer Experience: Explainable AI enhances the customer experience by helping businesses explain complex AI-driven decisions to their customers. Whether it’s a loan application or a personalized recommendation, customers value transparency, which fosters trust and loyalty.
Applications of Explainable AI in Business Intelligence
1. Credit Risk Assessment
In the financial sector, credit risk assessment is a critical process that involves evaluating the creditworthiness of individuals or businesses. Explainable AI can provide insights into credit decisions, helping financial institutions understand the factors leading to credit approval or denial. By providing clear explanations, organizations can ensure fairness, identify potential biases, and comply with regulatory standards.
2. Healthcare Diagnosis
In the healthcare industry, Explainable AI can assist medical professionals in diagnosing diseases and recommending treatment plans. By offering transparent explanations for diagnoses, AI systems can help doctors understand the underlying reasoning and provide patients with clear justifications. This promotes trust between doctors and patients and enables more informed healthcare decisions.
3. Fraud Detection
Explainable AI is highly valuable in fraud detection and prevention. By providing explanations for suspicious activities, AI systems can help organizations identify fraudulent transactions, understand the patterns that indicate fraud, and take appropriate actions. This transparency not only aids in preventing financial losses but also enhances customer trust.
4. Compliance Monitoring
Regulatory compliance is a vital aspect of industries such as banking, insurance, and pharmaceuticals. Explainable AI can aid in monitoring compliance by providing transparent explanations for decision-making processes. This helps organizations demonstrate adherence to regulations and standards, minimizing the risk of penalties and reputational damage.