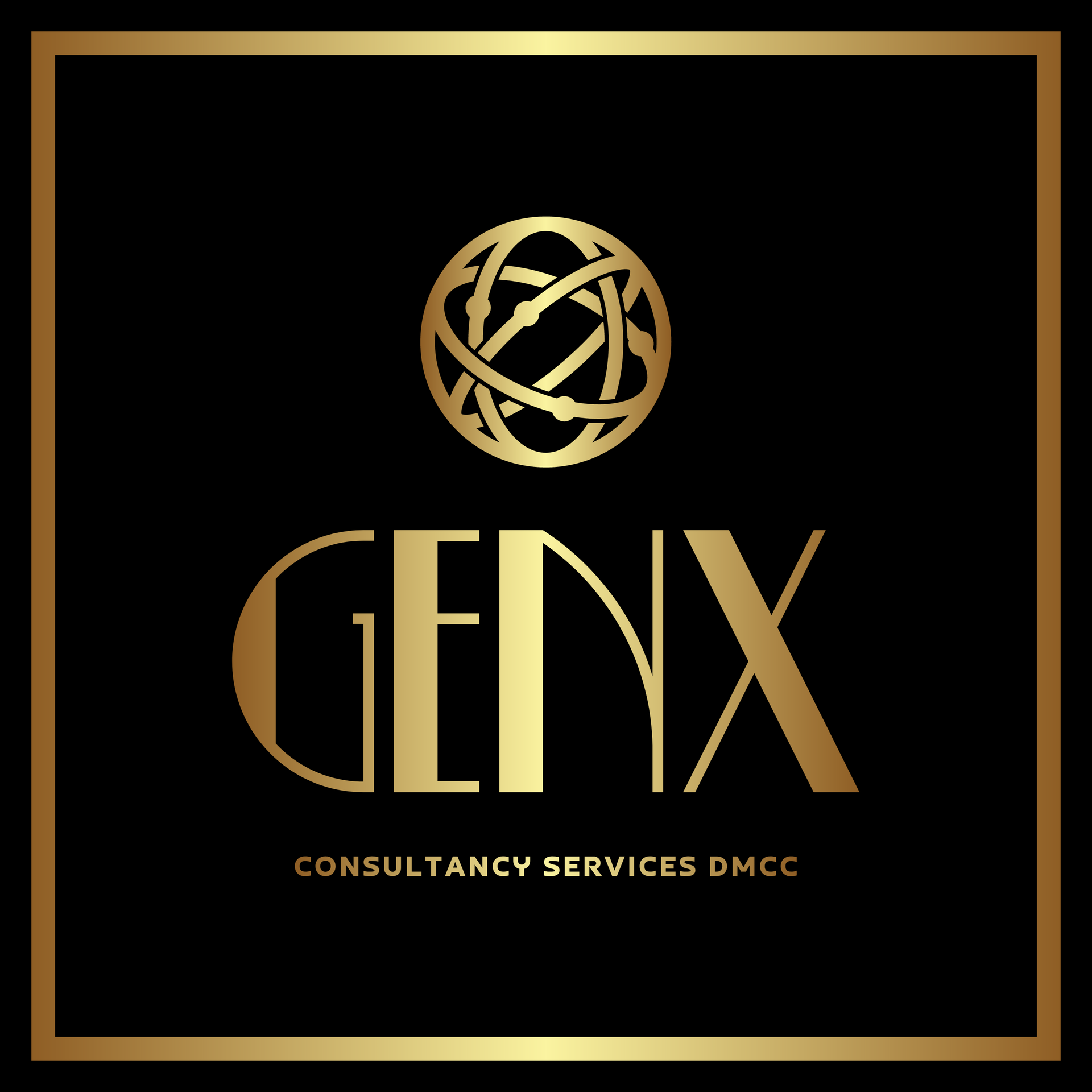
This is a subtitle for your new post
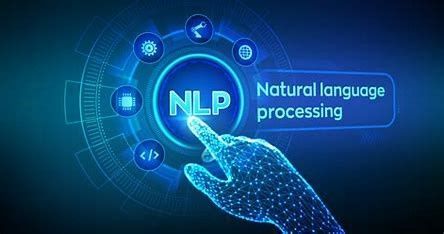
What is Natural Language Processing?
Natural Language Processing, as the name suggests, is a branch of Artificial Intelligence (AI) that deals with the interaction between computers and human language. It encompasses the ability of computers to understand, interpret, and generate human language. NLP enables machines to comprehend the nuances of natural language, including syntax, semantics, and pragmatics, allowing for more effective communication between machines and humans.
The Benefits of Natural Language Processing in Business Intelligence
1. Enhanced Data Exploration and Analysis
With NLP, businesses can effortlessly interact with their data by using natural language queries, eliminating the need for complex coding or query languages. Users can simply ask questions in their own words and receive meaningful insights. This democratizes access to data, making it more accessible to non-technical users and promoting self-service analytics.
2. Improved Decision-Making
NLP enables decision-makers to gain a deeper understanding of their data, facilitating more informed and data-driven decisions. Natural language queries can be used to explore patterns, trends, and correlations within the data, empowering organizations to identify opportunities, mitigate risks, and optimize their strategies.
3. Real-Time Data Insights
In today's fast-paced business environment, real-time insights are crucial for staying competitive. NLP allows for the analysis of data in near real-time, enabling organizations to respond quickly to changing market conditions and make timely decisions. Whether it's monitoring social media sentiment or tracking customer behavior, NLP can provide real-time insights that drive actionable outcomes.
4. Improved Customer Experience
NLP plays a vital role in enhancing customer experience by enabling more natural and intuitive interactions. Chatbots and virtual assistants powered by NLP can understand customer queries and provide accurate responses, replicating human-like conversations. This not only improves customer satisfaction but also reduces the workload of customer support teams.
5. Efficient Data Extraction
In traditional BI systems, extracting relevant information from unstructured data sources can be a time-consuming and error-prone task. NLP automates this process by analyzing text data and extracting key entities, sentiments, and themes. This enables organizations to gain insights from sources such as customer reviews, surveys, and social media posts, saving time and effort in manual data extraction.
6. Language Translation and Localization
As businesses expand globally, language barriers can hinder effective communication and decision-making. NLP technologies can break down these barriers by providing accurate language translation and localization. This enables organizations to analyze data, communicate with customers, and understand market trends in different languages, facilitating international growth.
Implementing Natural Language Processing in Business Intelligence
1. Define Clear Objectives
2. Choose the Right NLP Tools and Technologies
3. Data Preparation and Annotation
4. Continuous Model Training and Improvement
5. User Adoption and Training
The Future of Natural Language Processing in Business Intelligence
1. Conversational Analytics
Conversational analytics aims to go beyond traditional query-based interactions and enable more interactive and dialogic conversations with data.
2. Sentiment Analysis for Customer Experience
Sentiment analysis, a key aspect of NLP, will continue to play a vital role in understanding customer sentiment and perception.
3. Knowledge Graph Integration
Knowledge graphs, a powerful representation of structured and interconnected knowledge, can be integrated with NLP to enhance data exploration and analysis.
4. Multimodal NLP
Multimodal NLP combines text with other forms of data, such as images, videos, and audio, to enable more comprehensive understanding.
5. Privacy and Ethics
As NLP becomes more pervasive, ensuring privacy and ethical use of data becomes paramount. Organizations must prioritize data governance, transparency, and user consent to build trust and maintain compliance with regulations.